Big Data, IoT and SEEBURGER
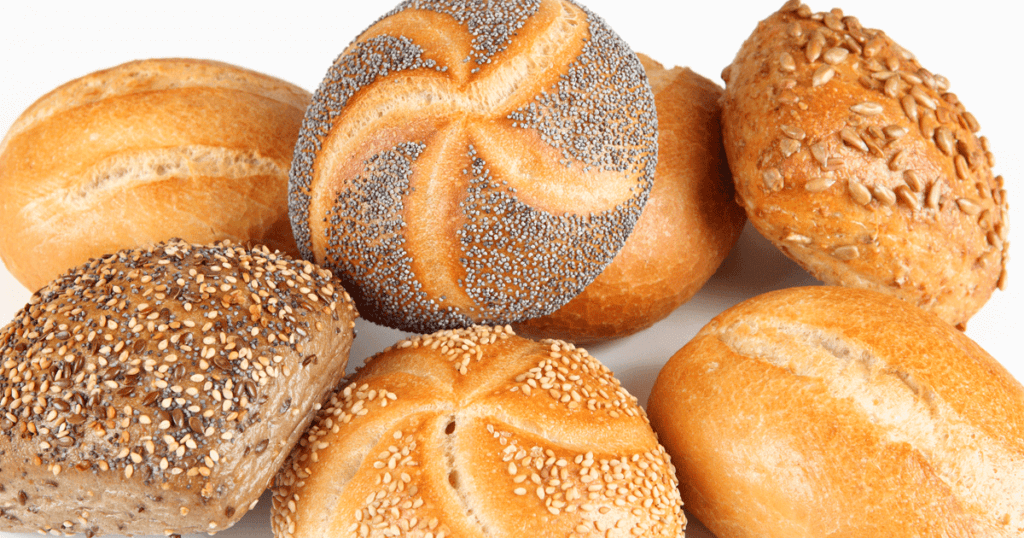
What do bread rolls and big data have in common?
Data is generated in masses – even in places where one would not expect it. The trick is to find ways and means to collect these unimaginably large amounts of data in one place and then to make them available from there as quickly as possible and use them for specific purposes. But what happens when you try and bring together much-discussed technologies such as IoT (Internet of Things), AI (Artificial Intelligence), Big Data, APIs (Application Programming Interfaces) and Data Lakes into a single scenario? Read on!
In order to plan how much oven-fresh supply of sesame rolls and pretzel sticks that have to be delivered to the sales points throughout day, the sales figures of the last few hours are transmitted in real-time from the cash registers to the head office. Additionally, aggregated historical sales data could be factored in to automatically forecast demand in the immediate future. This would optimally control the replenishment quantity. The same challenge arises with any type of consumption or dispatch quantities – not only in the retail sector, e.g. material flows within a company (intra-company logistics), container flows at transhipment points or even the traffic flows on which logistics processes are directly dependent.
It was the example of ‘traffic flows’ that was a fundamental catalyst for a research project at SEEBURGER. Utilizing Artificial Intelligence (AI) for the creation of automated forecasts based on aggregated mass data, the following scenario was created in SEEBURGER‘s Business Integration Suite (BIS) with various open source solutions:
Data traffic taken quite literally – traffic as a data source
This research project dealt with data traffic, APIs, data lakes and AI. The objective was to gain new insights into IoT use cases (processing of sensor mass data) and to explore AI approaches.

At the beginning of the project a very basic question arose: from where do you acquire the huge amounts of data in real-time? The researchers literally found what they were looking for on the road – at the German Federal Ministry of Transport and Digital Infrastructure. There, real-time data on traffic flows on German motorways is made available, which can be logged and accessed by each federal state. The data is collected via sensors along the motorways and aggregated in pools by the traffic control centres. For example, traffic speed and traffic flow are measured for each lane and for each type of vehicle.
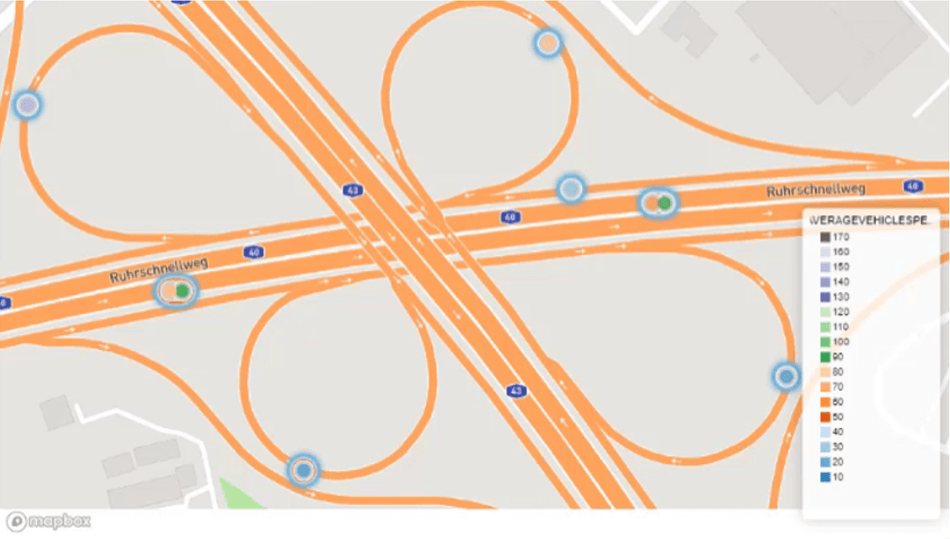
For their research project, the R&D department at SEEBURGER utilized the real-time data of two German federal states at the mobility data marketplace. Specifically, it was the aggregated data of the past minute that was accerssible. This data was regularly retrieved and consumed by the SEEBURGER Business Integration Suite (BIS) via the API of the mobility data marketplace.
To analyze and process this data in a unified way, it first had to flow into a data lake from where it was collected – providing access to a continuously growing pool of real-time data that spanned several months. Making this data usable for Big Data tools, it had to be converted into appropriate formats and transferred to other systems.
- The SEEBURGER Business Integration Suite (BIS) provides these capabilities.
In this way, for example, all kinds of streaming dashboards can be created that perfectly reproduce the real-time movements of traffic. But there is more to it than that. With a data stream of this nature, a lot more can be achieved:
Machine Learning and AI
Feeding this data stream over the course of several months for training purposes into a system such as Tensorflow, which is possible via the SEEBURGER BIS, these systems can predict traffic speed and traffic flow data for each lane and for each vehicle type. Very good results have already been achieved for predictions of the average traffic flow 30 and 60 minutes ahead, as illustrated in the diagram below. For this purpose, a black line which represents the forecast for the next 30 and 60 minutes, was respectively placed over the blue zag line that represents the actual measured traffic flow.
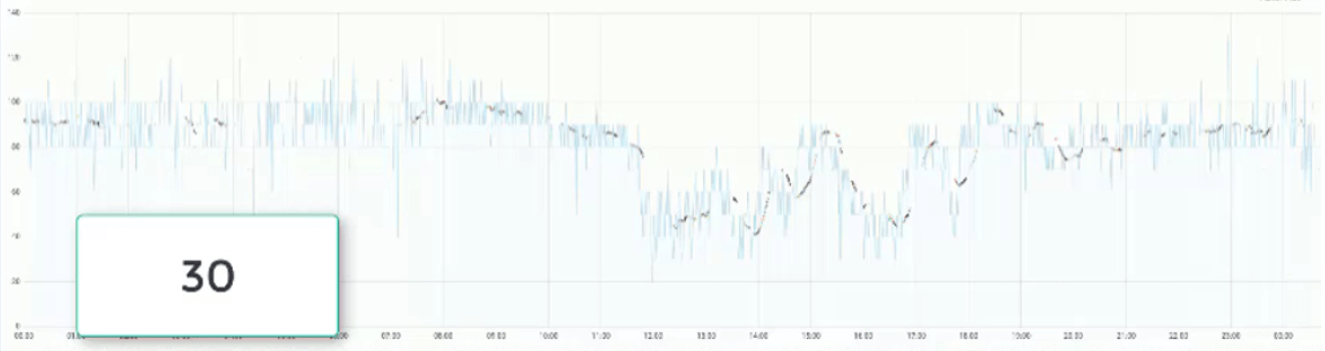

A look into the future
This type of predictive capability can be utilise in many ways. From traffic jam forecasts to route planning to the possible realization that one or the other section of the motorway needs to be extended – there are no limits.
We at SEEBURGER are also considering how such capability can be made available to SEEBURGER customers. The day-to-day operations of many of our customers are highly dependent on external factors such as traffic flows. These dependencies become more and more critical the more the operative day-to-day business is endangered by disturbances such as traffic jams. Forecast data on traffic flows, as described here, could help to cushion these disruptions.
This is what happens when technologies such as IoT (Internet of Things), AI (Artificial Intelligence), Big Data, APIs (Application Programming Interfaces) and Data Lakes are brought together. The basic prerequisite is that the data that already exists anyway, is integrated and thus made reusable for multiple purposes.
Thank you for your message
We appreciate your interest in SEEBURGER
Get in contact with us:
Please enter details about your project in the message section so we can direct your inquiry to the right consultant.
Written by: Dr. Johannes Strassner
Dr. Johannes Strassner, Head of Research, is responsible for application-oriented research on innovation of SEEBURGER's business integration technologies and solutions. The current focus is on Machine Learning, Big Data, API, Blockchain and IoT. Johannes Strassner received his doctorate at the University of Koblenz-Landau for his work on ‘Parametrizable Human Models for Dialogue Environments’. He joined SEEBURGER in 2011 after research activities at the Massachusetts Institute of Technology and the Fraunhofer-Gesellschaft.